Data is pivotal to organizations in today’s digital world because it provides valuable information that drives economies and business decisions.Every business needs a well-organized data infrastructure to fully harness data resources, maintain data flows, minimize redundant data, protect sensitive data, and preserve data quality.
Data infrastructure is a collection of various assets, including services, policies, networking, hardware, and software, that enable data sharing, consumption, and storage. In other words, data infrastructure is the correct combination of process, technology, and organization that provides the foundation for enterprises to create, manage, secure, and utilize their data. That’s why organizations must develop and run efficient data infrastructures. With such a structure in place, the right set of data will reach the right systems or users at the right time, and there’ll be more effective data-driven decision-making.
Also, strong data infrastructures help enhance the productivity and efficiency of work environments while increasing interoperability and collaboration. Ultimately, organizations are able to boost supply chains, lower operational costs, and maximize customer data for better engagement. Keep reading to discover the steps for building a data infrastructure for your organization.
1. Map Out Your Strategy
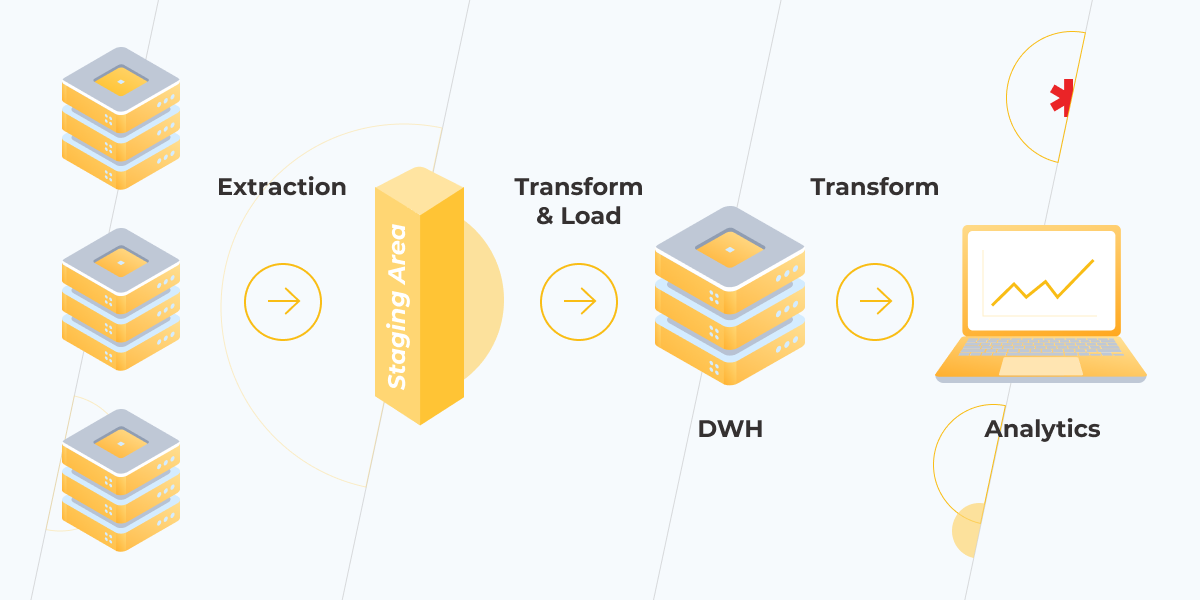
You need to perform a detailed data audit during which you’ll decide what data to collect, where your data will come from, how it’ll be secured, and where you’ll manage the data. You can manage your data on-premises through your servers or in the cloud via a cloud-hosting service. When developing your data strategy, you should also decide who needs access to your data and why. Defining your data infrastructure strategy early on will save you significant effort and time in the future.
2. Choose a Data Repository
There are various types of data repositories available in the market. You need to decide what repository to use to collect data and where to house it. The three primary types of data repositories are data lake, data warehouse, and hybrid. Data lake repositories are often used to store raw, unstructured data, whereas data warehouse repositories are mostly for structured, filtered data. In the hybrid approach, the data warehouse and data lake elements are combined.
With the multitude of data generated daily, developing a data warehouse solution will require more time and effort. However, you can invest in resources to enable you to build a data warehouse that contains well-structured data that is easy to analyze. The simpler architecture of the data lake makes it suitable for big data typically encountered by data scientists. One factor to bear in mind when deciding between data lake, data warehouse, and hybrid is that they use different technologies. Data lake primarily uses NoSQL, whereas data warehouse utilizes SQL.
3. Clean and Optimize Your Data
Inaccurate data can negatively impact the different departments of an organization. So, you need to prioritize proper data cleaning when developing your data infrastructure. This is especially crucial if you’re creating a data warehouse. Your goal should be to ensure that your data satisfies specific conditions, including completeness, consistency, uniqueness, timeliness, and accuracy.
To properly clean and optimize your data quality, you should identify and delete duplicate datasets and other irrelevant data. Next, fix errors in your data structure and develop rules for cleaning incoming data for your entire organization. Consider investing in tools that will enable you to clean your data in real time.
4. Develop Your ETL (Extract, Transform, and Load) Pipeline
As the name implies, ETL is the process of extracting, transforming, and loading data from multiple sources to a unified data repository such as a data warehouse or data lake. An ETL process is crucial to data warehousing and analysis because it brings structure, clarity, quality, velocity, and completeness to the data sets. Ultimately, your data becomes more organized and more accessible. RudderStack, one of the customer data platform companies, points out that ”you can effortlessly connect your data stack from real-time streaming to ETL and reverse ETL, which gives you complete visibility into your customer data flows.”
When working on your ETL project, you need to consider data scaling and address broken data connections, contradictions between systems, and the likelihood of your data formats changing over time. So, you must anticipate future data needs in the process. There are various tools that can help accelerate, automate, and handle various aspects of your ETL process. Try to research any tool you’re considering using for your ETL pipeline before acquiring it.
5. Implement Detailed Data Governance
- Business
- Crypto
- Digital Marketing
- Education
- Fashion
- Games
- Health
- Home Improvement
- Mobiles & Apps
- News
- Software
- Sports
- Tech
- Technology
- Uncategorized